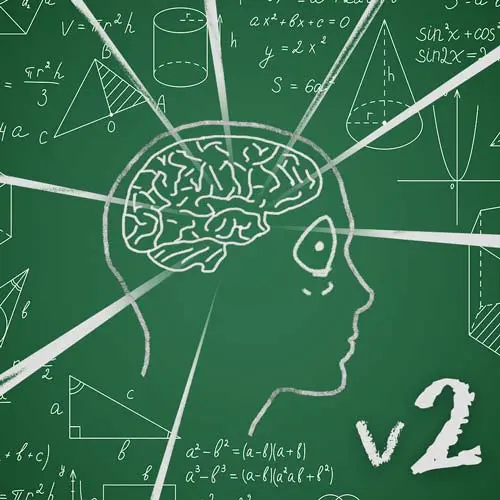
Check out a free preview of the full The Last Algorithms Course You'll Want (Part 2) course
The "Max Subarray" Lesson is part of the full, The Last Algorithms Course You'll Want (Part 2) course featured in this preview video. Here's what you'd learn in this lesson:
ThePrimeagen introduces the concept of finding the maximum subarray within an array. They explain a naive solution that has a time complexity of O(n^3) and then propose a more efficient approach using dynamic programming. They walk through an example to demonstrate how to use previous values to compute forward values and emphasize the difficulty of solving dynamic programming problems.
Transcript from the "Max Subarray" Lesson
[00:00:00]
>> Now, right now, this is not really great problems. I feel whenever I see these, it doesn't hit home. So we wanna take it up one more level. We're gonna do the maximum subarray. So this is like a classic interview debacle, where you are asked to find the maximum array within an array.
[00:00:19]
So an array can look something like this. 3, -4, 1, 2, -1, 5, -7. The maximum subarray is very obvious, 1, 2, -1, 5. Add all those numbers together, that is your largest contiguous subarray. Now a naive solution would to do something like this, sum, sum, sum, sum, sum, sum, sum, sum, step forward once, sum.
[00:00:50]
Now we're on the -4. Then we go all the way again. Right, we're gonna do that every single time, just trying to find the maximum subarray. Computing the same value though many, many times throughout this over and over and over again. We are gonna do an n squared walk here and we're gonna do that n times.
[00:01:09]
So it's an n cubed algorithm, which is just like that's just not what you want in your life. This is almost always a bad plan to do n cubed. Unless if you're multiplying matrices. Then you need a degree in physics, and then you can somehow get it to be less and there's like crazy optimizations and somehow everything works out and it's fast.
[00:01:27]
All right, so how do we do this? Well, let's think about it in a different way. At this point, we know the maximum value, right? So we start out at 1. One the maximum value no matter what. If you have an array of one, the maximum value is just that first element.
[00:01:43]
So right now we have a max value of 3. We also have a current sum of 3, right? All right, let's walk forward once. Is our current sum negative? No, therefore, let's add this number to it. So current sum becomes -1. Is -1 bigger than our maximum value.
[00:02:12]
No, therefore, our maximum value remains as 3. We go here. Is our current sum negative? Yes it is. We found a spot where the maximum value goes below 0, therefore it just can't be. We're in a bad spot, we just ignore it, right? And so therefore we're gonna reset current sum to the current node.
[00:02:33]
It's not 1. Is one less than the maximum value? Or sorry, is 1 greater than the maximum value? No! Leave it alone. All right, go to 2. Is current value or current sum less than 0? Is it negative? No, therefore, add that number to our current sum. All right, is this larger than our maximum value?
[00:02:59]
No, therefore, we move forward. Is our current sound less than 0? No, it's not add that to it.1 is it greater than our maximum value? No, therefore move forward. Is this less than 0? No, it's not therefore add it. We are at 6. Is this greater than or less than our current maximum value?
[00:03:19]
It's greater than. Update your maximum value. Now we go forward. That's right here. Is this thing less than 0? No, it's not. Now it's a -1 cuz we added it to it. Is this greater than or less than our maximum value? No, it's not our maximum sub array is a value of 6.
[00:03:35]
If we just do it with our eyes, that's 1 + 2 + -1 + 5 or simply 4, that is a 3, 3+4, that's 7, we got it. All right, fantastic, wait.
>> [LAUGH] You-
>> Did I do terrible? I did do terrible math. I went 1 to 2, didn't die, and then I went back to 1.
[00:03:53]
Okay, it should be a 3. Okay, no one's, and I said quick math today. Okay, my quick math's not that great today. Okay, quick math. You get the idea though. That is how we are using previous values to compute forward values. We're taking it and we're walking across this and doing it.
[00:04:10]
Now if this doesn't make it clear, we have one more problem that should make it really clear how to think about these. Now, how you think about these and how you come up with dynamic solutions is incredibly difficult. Anyone that's just like, dynamic programming is easy. I'm like, no, it's not, and you're being jerk because it's not easy.
[00:04:26]
It's crazy. It's like I only know how to solve dynamic problems because I know how to solve those problems already. It's very hard to see a new one and just be able to do it really quickly.
Learn Straight from the Experts Who Shape the Modern Web
- In-depth Courses
- Industry Leading Experts
- Learning Paths
- Live Interactive Workshops