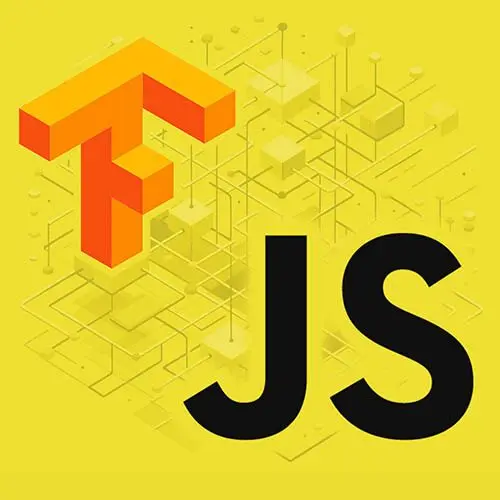
Lesson Description
The "Saving the Model Data" Lesson is part of the full, Machine Learning in JavaScript with TensorFlow.js course featured in this preview video. Here's what you'd learn in this lesson:
Charlie writes the model data to a directory in the project. The program is executed a few times to see if the accuracy improves. Additional model directories can be created to save several versions with varying accuracies.
Transcript from the "Saving the Model Data" Lesson
[00:00:00]
>> So here, hopefully it has worked for you. If you've run node train-drawings.js, you could you should see this this as well in the terminal. And if we go back to the code, what we haven't done here is we haven't actually saved the model. So here you don't see any model folder where the model has been saved, so we can write this now.
[00:00:23]
So here, let me just check this second. Okay, so we can do a wait and then model.save, so that's the method that you can call to save your model locally, and as we're going to save it in as a local file, we can write file as a string.
[00:00:46]
So, file:// and then we're going to have it under the public folder and we're gonna call it model, and that should be it. So that means that whenever the training process is done, it will save our model into a new folder that is going to actually, don't remember if it creates the folder directly or if we're going to have to credit ourselves, but we can try that just now.
[00:01:13]
So if you save your file and we run it, might complain about, I don't know what the model folder is, no, it didn't complain? It did. Okay, so it does create automatically the folder that as long as you pass the right path to it. So now it means that here we have our machine learning model that has very poor accuracy [LAUGH], but it means that here you have, as I was talking about yesterday.
[00:01:40]
In the model.json file, you have the details of the layers that we created ourselves, so we can see our sequential, here we have a first layer that's a conv2d. And then there are probably some default settings because variance scaling, we didn't write that, so it means that that's probably one of the default stuff.
[00:02:01]
And here we have a kernel_size that we did define, we didn't define strides. I'm not sure how to explain what strides are, but here I think the default values will be 1,1. And here we have a MaxPooling2D layer, and you have our activation that's using 10 units. So here you can see that you have a mix of things that we have cleared manually and a bunch of things that are probably have default values.
[00:02:26]
But here we have this and we should be able to also see that it's actually then requiring the weights that been filed and if you remember from yesterday, that's like a binary file so you can't really exactly see what's going on. But the binary, the weights file, it contains the way that the model was tweaked as it was being trained.
[00:02:46]
So usually, sometimes if you only know the layers of a model, that doesn't necessarily tell you really that much. I think at the moment, if you follow a little bit what's going on with AI and open AI and things, you can sometimes hear people being, open source the weights.
[00:03:03]
And it's because if you know the way that your model was created in terms of layers, it doesn't really tell you everything. It's like the weights is more the way that it was tweaked to get to that, either accuracy or things like that, so that's also important. And I guess usually maybe companies don't want to open source that okay, so now we have our model and we saved it to our folder.
[00:03:30]
So maybe at this point, before we use it in our app to actually predict things, let's maybe try to make some changes and see if we can update the accuracy. So the thing as well as, you might have noticed that is that I got 0.5, but the thing is sometimes you can rerun it and it will actually give you see all of a sudden have 0.9, [LAUGH].
[00:03:53]
So, the thing as well is that with machine learning models, sometimes they're people you will hear people tell you that they're some kind of like a black box you don't really understand how? They ended up making certain decisions or having a certain level of accuracy. So unfortunately, sometimes I just ruin it a few times and when the accuracy is good, then I stop touching it, which is probably not the best way to do it.
[00:04:15]
But it's also in AI, you'll hear people say that, if companies use AI, you should get to a point where you want to be able to explain how the model made certain decisions, but it's difficult. But I think here I have no idea what all of a sudden, it's 0.9 but it means that if I did not retrain it now and I used the model that is now in my model folder here, it means that in the UI I should expect it to recognize better a circle and a triangle.
[00:04:45]
So maybe, for me, maybe we're not gonna tweak it now, maybe I'm gonna use mine that's on 0.95 now. So it means that 95% of the time it was able to actually recognize correctly what was in the test folder. So if for you it's like a lower accuracy of below 0.5, maybe like retrain it a few times.
[00:05:06]
And then if really it's the next step of this project is we're going to use it in the UI to get live data. And then once that's working, then we can maybe tweak parameters or add a layer and see how that works as well. But here if we look at look at the output of the model, we can see that the values do change over time.
[00:05:25]
So here I started at 0.653, and then it kind of well, the next step was a bit lower. And over time, you can either increase the number of epochs or increase the batch size or add more images, so there's a lot of things that you can do there.
Learn Straight from the Experts Who Shape the Modern Web
- In-depth Courses
- Industry Leading Experts
- Learning Paths
- Live Interactive Workshops