Predicting, Saving & Loading Models
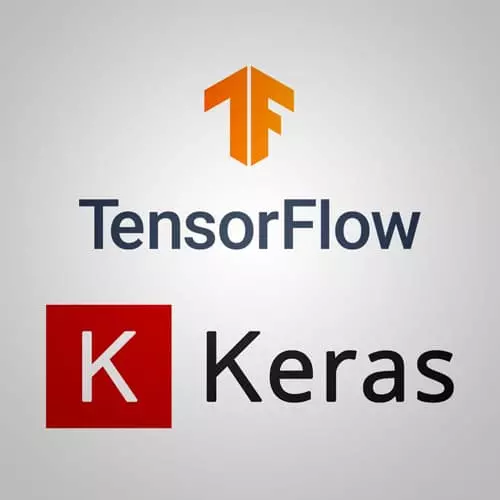
Check out a free preview of the full A Practical Guide to Machine Learning with TensorFlow 2.0 & Keras course
The "Predicting, Saving & Loading Models" Lesson is part of the full, A Practical Guide to Machine Learning with TensorFlow 2.0 & Keras course featured in this preview video. Here's what you'd learn in this lesson:
Vadim provides different steps that one can take after training a model, accesses individual layers, starts predictions, and visualizes neural networks.
Transcript from the "Predicting, Saving & Loading Models" Lesson
[00:00:00]
>> The only thing I wanted to show you right now we played around with different neural networks right with fully connected neural network with the convolutional neural network to recognize those seizures. But I haven't actually show you what to do with the model after you've trained it. So after you trained the model, there are actually a couple of things you can do.
[00:00:25]
For instance, let's quickly see if I have it here. If not, then I will, you can access individual layers, right? And for instance, you can get layer by the index or just go through the weights with corresponding index which simply gonna to be the number of the layer you trying to access to you.
[00:00:53]
And yeah, you can even do the predictions. So for instance, if you just do the model.predict and provide the input. The only thing with the images remember that's our image it was simply 28 by 28 pixels, right? But our model takes several of images when we're training so we need to reshape it.
[00:01:22]
So basically in create list which will contain only one image. But if we create several lives, we can create. For instance, if we want to process multiple images simultaneously, we can just create the list of those images and then do model predict and provide this list and as the results, our model will create predict what it's actually observing.
[00:01:46]
And you always can do a saving so model is it safe? I think I already terminated this notebook. Yeah, sorry about browsing. Let's quickly just restart we initialize the green data and our model. And we can even quickly train it, as far as I remember, the training was pretty fast.
[00:02:22]
And by the way, yeah, if you want to visualize the topology of your neural network. [LAUGH] This one is actually wrong. You can simply use plot model from TensorFlow Kerris utilities utils. It gives you a pretty nice graphic and you can save it into file, PNG. Or if you want a higher resolution vector graphics, you can even use SVG format.
[00:02:53]
Just pretty good if you will want to use kind of visualize the topologies, right off your neural network. Once again, a model summary works as well. So, let's quickly just retrain our model. This one should be pretty fast. And as I said, if you want to, after you're done training, just save you model, what you can do, you can use model, I'll just wait for it to finish.
[00:03:27]
Or actually let me interrupt, reduce. We don't need 40 epochs here we need probably only 5 and they can restart the wrong. Let's see, we're already actually it's 99% accuracy. So I think we good and it's with fully connected neural network it's pretty, pretty small and how many layers we have here only one hidden layer right?
[00:03:55]
The last dense layer we can think about it as the just the player with the neurons which will be activated depending on which digit we're actually looking at. And if you want to save, You can do save the model completely, in this case it will save the topology itself.
[00:04:18]
And yeah, we can just specify by a file path or just the name for instance model.h5 or something like this. If we execute it, it will save the file it will also appear as a modelh5. So all the weights and topology of the model will be simply stored in this file and we can even load it from a new notebook.
[00:04:49]
If we just for instance, open a new notebook and say, although I have to now, for the model, let's actually return back and just use the new cell here, just because my Kerris is already implemented here. So I can do something like this model load. And I can only load weights.
[00:05:14]
So I should do save weights in this case. Save weights and then the model will have to be recreated with all the layers used during the training. But then I can simply load weights from the file. Right, and for instance if you don't want to spend time retraining your model every time you can just dump those weights on your file system and download them whenever you need them.
[00:05:45]
But the initialization of the model itself, so model definition have to be. I have actually two models here, right, here and this one. But anyway, you got the idea is the way how you can train it, save it and then use it for later on and doing the prediction as I said, it's pretty, simple.
[00:06:14]
I've showed it right here. So first you just reshaping your image so it was, let me actually do the explicitly. So if we printix_text (0) shape and print our input shape. You'll see what happened. So original image was only 28 by 28 pixel but I just grabbed this image and by reshaping means that I put it inside another list.
[00:06:56]
That's it. And just what's our model expect to be the input to do the predictions. And then if we print the results of the prediction we should see this 10 different numbers. That's the simply getting the signal coming out of all our 10 neurons, right? And actually the number we're looking at is number 7.
[00:07:25]
So if we calculate 0, 1, 2, 3, 4, 5, 6, 7, that's the highest number among those. So you can see all of those have minus. 7 power minus 10. And this one is pretty close to 1. So our model is super confidence that we're looking at seven and you can even visualize those distribution.
[00:07:47]
So you see we have the peak seven and the rest is pretty small. So, yeah and that's the number we're analyzing, number 7.
Learn Straight from the Experts Who Shape the Modern Web
- In-depth Courses
- Industry Leading Experts
- Learning Paths
- Live Interactive Workshops