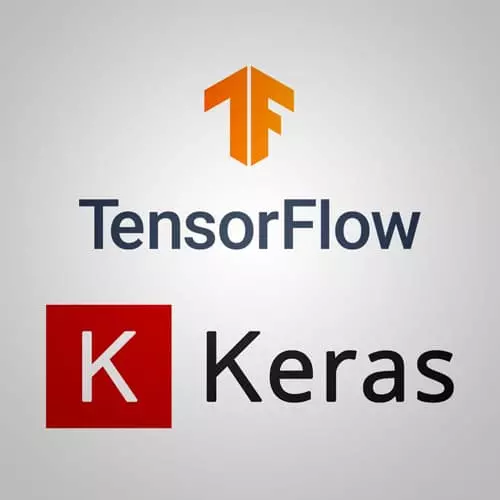
Check out a free preview of the full A Practical Guide to Machine Learning with TensorFlow 2.0 & Keras course
The "Plotting Uniform Data" Lesson is part of the full, A Practical Guide to Machine Learning with TensorFlow 2.0 & Keras course featured in this preview video. Here's what you'd learn in this lesson:
Vadim demonstrates how to use the Matplotlib library to depict uniform data. The following sections iterate over the same linear regression model.
Transcript from the "Plotting Uniform Data" Lesson
[00:00:00]
>> We already dig quite deep into architecture of, [LAUGH] TensorFlow. But it kinda beats the purpose of the introduction to machine learning, right? So once again I wanna return back and tell you about Linear regression. And so it's almost like a grandfather of your neural networks, so I want to show you how you can actually tweak your weights and biases to get to the optimal value.
[00:00:35]
So what we will do, is simply first let's create the data, the data we will be using to to learn something about this data distribution. So we will be using tensor flow random to add a little bit noise to our data, and let's just define the function. Define let's say data creation.
[00:01:05]
And what we can do, we can just provide here the values we will try to find. So for instance, our weight for now, let's just keep only one weight and one input signal, right? So for instance weight, let's say equal to 0.1. Our bias, let’s say equal to 0.5, why not?
[00:01:30]
And then in our function what we will try to do is just, first generate x, and that’s gonna be our = data. So I want to randomly generate it, so I will use tf.random.uniform. If only I can type. How much data points do I want? Let's say, shape = (), I can also specify it as the parameters, so n=100, for instance.
[00:02:09]
So let's just print X, just to see what it's gonna create. I haven't called my function yet, right, so let's actually call it here, data_creation. And in Python, if hose arguments were provided. So if default values will be provided, they will be used in the function body, but I can modify them.
[00:02:34]
So for instance, if I explicitly say I want my wb = now to 0.5, I can do that here. And if I don't specify anything else, then a default value b will be used default value n. So what happened here, we printed out X and in our X we have just 100 numbers uniformly distributed between 0 and 1.
[00:03:07]
To show you that it's truly uniformly distributed, what I can do, I can just plot those numbers. So, let's say X =, let's return back to our original value. So, new Tensor is created, what we can do we can just use matplotlib inline. If you're once again not familiar with the Jupyter Notebooks, this percentage in front of the line means that I'm using Magic Word.
[00:03:41]
In Jupyter lingo it means that I want to run some internal commands which Jupyter Notebook will recognize. And, this particular command matplotlib inline means that all the images created by the matplotlib library. Will be printed out on screen in the Jupiter Notebook. A matplotlib is actually a super nice library for visualization.
[00:04:08]
If you go to matplotlib.org, you can find a lot of useful information about this library, as well as really nice examples. That's what I usually use, I just kind of go to the examples. Look at the Graphs I want to draw, what kind of similar graphs I want to draw.
[00:04:28]
I just click on them, and that will give me an example of the Python code which I can then just adjust to my purposes. So you can say this library is pretty awesome.
Learn Straight from the Experts Who Shape the Modern Web
- In-depth Courses
- Industry Leading Experts
- Learning Paths
- Live Interactive Workshops