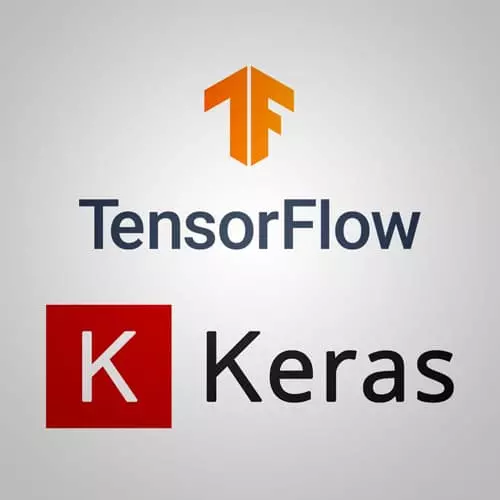
Check out a free preview of the full A Practical Guide to Machine Learning with TensorFlow 2.0 & Keras course
The "Long Short Term Memory" Lesson is part of the full, A Practical Guide to Machine Learning with TensorFlow 2.0 & Keras course featured in this preview video. Here's what you'd learn in this lesson:
Vadim explores Long Short Term Memory or LSTM ,one of the classes of neural networks , explains how one has to build intuition when working in machine learning and deep learning, highlights the importance of keeping a clean training dataset, and trains a model that recognizes the author of a quote.
Transcript from the "Long Short Term Memory" Lesson
[00:00:00]
>> Here, what we're are doing, we once again, what we will do, we will use LSTM. LSTM stands for, Long Short Term Memory, it's one of the modes in the class of recurrent neural networks. And in this example we'll just grab texts by different authors. There'll be Butler, we can use Shakespeare for instance, right?
[00:00:26]
Yeah, I think I added Shakespeare at the end. I wanted to actually have fun with this example and download lyrics by Eminem, Back Street Boys and who else, Britney spears. And just compare if we can distinguish based on lyrics between those singers, but unfortunately, website with lyrics it was down yesterday.
[00:00:53]
So were not able to prepare this demo, but we will rely on just authors. There is a lot of Shakespeare, Butler, Derby, and Couper. So, what are we doing here it's kinda another thing that's more advanced example. Because we will have a particular buffer and we'll specified batch size, and all of those can be hyper parameter and will definitely affect the speed and accuracy of our model.
[00:01:25]
So we can just maybe later play around with to get better results, but we're loading and shuffling our data with the buffers. And you can see that the text we have, for instance, that's not, blah blah blah anger and yawn and at least those both texts belong to the same author.
[00:01:48]
But for instance, this one is a different author and index 2, I think that's my, so 0, 1, 2 it's Batler. But you got the idea, is basically phrases which belong to different authors. And what we were trying to do, we were trying to train our neural network to recognize which author wrote a particular phrase.
[00:02:18]
So tokenization takes a little bit of time. And what tokenizer is doing is just grabbing this raw text, finding the kind of putting it in the database of words and then assigning index to all of those words. And you can see that we have 23,000 unique words in our vocabulary, which is quite impressive.
[00:02:46]
All right, and now our phrases, for instance, that was, the example I grabbed was the first text. So the original text was just this phrase, it was reduced to the least of those corresponding indexes. All right, and so what we can do just prepare the data sets. Once again, can just use the padding, because we have phrases with a different length, right?
[00:03:19]
So what I can do, I can just simply say that I will be using 16 words. And if for instance we have less then we will populate first whatever kind of cells with my indices and the rest will be equal to zero. Meaning that those words were not present.
[00:03:41]
And if the text line I'm trying to feed there have more than 16 words, I will simply ignore the rest, I will only consider the first 16 words in the phrase. And the neural network is defined like this, we're still using sequential. First we're use an embedding with 64 degrees of freedom and bidirectional LSDM.
[00:04:09]
Basically will allow us to memorize the position of the words and then we can have, for instance, couple of dense layers. So I'm just gonna be having 64 and for instance in last layer we can have, I don't know, 16 for instance, right? So let's just run this model definition, a compilation we'll use Adam optimizer and sparse categorical crossentropy will be our loss function.
[00:04:36]
And it will take about four minutes to run, but I can probably just execute this and talk a little bit more about things you can do with text. So, Yeah, it's taking some time probably just to load all the data into the memory. Yeah, and it will be running only for three epochs, but each epoch probably will take about a minute or so.
[00:05:09]
All right, I'll just wait for it to finish, and I think that's gonna be the last notebook. So as soon as we get to this example we can play around a little bit and that's gonna be it, gonna be done, yeah. Right, so training is continuing on and you can see that it's our accuracy.
[00:05:42]
Accuracy on the training data set is already at about 77%. And for the, [LAUGH] validation accuracy for some reason it didn't calculate it, it's here. I'll say maybe if we'll successfully calculate it on, it's actually doing it right now. Interesting, interesting, so for the validation data set, the accuracy, it's calculated was 87% not bad.
[00:06:18]
>> What would you consider bad.? You've been saying not bad and what seems like random numbers to me? Is it just because-
>> The thing is when I played with the text analytics, quite often I was getting something close to 60%. And having almost 90, for me it's not bad.
[00:06:42]
It's just yeah, that's actually pretty interesting point. I consider machine learning and deep learning not to be a science at this point but more like an art. And quite often you almost like need to build an intuition what works and what not working for a particular type of problem.
[00:07:05]
Unfortunately, me telling you that you need this particular number of neurons in your layer and you want to use full, well, maybe you can say that fully connected convolutional neural networks can be used for these particular types of problems, right? And recurrent neural networks can used for text, is just because we want to remember the position on the words.
[00:07:23]
But at the same time, all other parameters can, how many neurons you want to have in the intermediate layer. All of that almost kinda comes with the intuition and learning which tricks works also heavily depends on your training data set. Because really good phrase, I like it a lot, you are what you eat.
[00:07:45]
It exactly refers to neural networks. The thing is if your data set is polluted, if it's not preprocessed properly, most probably your neural network will learn something ridiculous. For instance, if you have commas, two commas, then you comment probably gonna be negative. It's not true, right? But somehow neural network might figure out this dependency just because you had more examples which were negative, which had two commas in it.
[00:08:16]
It's ridiculous example, but it actually what's happening in real life. So you just need to play around with different techniques, even different activation functions and almost like a build intuition, what to expect depending on the model you having. And once again, as soon as even the same type of problem but different data is applied, most probably you will have to kinda reiterate once again and play around with different models and different setups and play around with different parameters.
[00:08:49]
So on top of choosing different topologies, you have different activation functions getting inside of those topologies and different structures. But also you have different optimizers, different lost functions, and different hyper parameters which control all of those. So you have so many degrees of freedom and unfortunately, all of that is still heavily depend on the data you're using for training.
[00:09:16]
So, that's why I'm saying it's not a direct science at this point. It's more like around building the intuition and knowing what worked for you previously and probably you should start from this point. But still to get better accuracy for your model most probably you'll have to tweak a little bit your hyper parameters to get to this sweet spot where the model will be performing the most.
[00:09:38]
And especially with the text, a lot will depend on preprocessing your text. How you build, how you transform your raw text into numbers. All right, we pretty much done, yeah, it took almost three minutes. So our evolution, we're doing it again. So we get to pretty close to 80% and we can even try our examples.
[00:10:05]
So, yeah, that's the phrase which converted to a particular index words and we can do the prediction. And with pretty high accuracy, my model is saying that that's the phrase was written by Shakespeare. Right because, do you want to try something else. Give me some very well known Shakespeare phrase
>> To be or not to be?
[00:10:31]
>> Good, okay.
>> [LAUGH]
>> I have no idea, will it work or not? Yeah, it thinks we have 70% that it is Shakespeare and yeah, about 16% that it was, what was the author number two? Author number two was Derby.
Learn Straight from the Experts Who Shape the Modern Web
- In-depth Courses
- Industry Leading Experts
- Learning Paths
- Live Interactive Workshops