Jupyter Notebook & TensorFlow
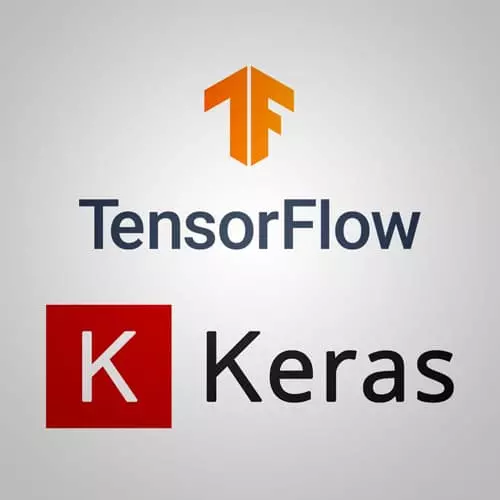
Check out a free preview of the full A Practical Guide to Machine Learning with TensorFlow 2.0 & Keras course
The "Jupyter Notebook & TensorFlow" Lesson is part of the full, A Practical Guide to Machine Learning with TensorFlow 2.0 & Keras course featured in this preview video. Here's what you'd learn in this lesson:
Vadim introduces Jupyter Notebook, and demonstrates how to access local files on the platform.
Transcript from the "Jupyter Notebook & TensorFlow" Lesson
[00:00:00]
>> All right, so we will be using Jupyter notebooks in our class today at Jupyter. To start it's if you're just type jupyter notebook, and dot, which corresponds to your local folder. It's where the Jupyter will be running the notebooks from. And Jupyter is the web server on one part, right?
[00:00:23]
So as you can see, it started on my local machine on the ports 8888 so I can access it through the browser by using localhost the rest. It also means that I can run Jupyter notebooks somewhere remotely on remote machine. And if the ports are open, I can simply access this Jupyter notebook server on any remote machine.
[00:00:50]
But that's the one parts, right, the frontend. On the backend what's happening is kinda interesting. It's actually running Python interpreter or several of them. And whatever code I will be running in the notebooks, it will be transferred to the Python interpreter and it will be executed on that machine where the Jupyter is running.
[00:01:10]
And if you, for instance, want to bring something out, it will be printed out back to the Jupyter notebook. So for instance to demonstrate, let's just start with some linear regression, right? That's the first example I have in my notebooks. So, as you can see, it basically allows me to create this interactive environment where I can just plot different graphs.
[00:01:33]
Or I can even text, well, put some text in mark down format. So, for instance, if I say this is list or something like that, or for instance, 1, another, an. [LAUGH] something like that. It will actually format the list. It's interesting, it's actually supposed to put numbers here.
[00:02:00]
If we do something like this, or second chapter, Yeah, it automatically will put it in numbers. It's mark down format, but it allows me to put some text as well as just run commands. So for instance, import tensorflow tf and print(tf.__version__) will just import the TensorFlow into my environment.
[00:02:28]
So it will be executed by the interpreter, and it will just preserve the state so I can run this command cells sequentially or in any order I prefer, right? And to execute them you just select the cell and press Ctrl+Enter or Shift+ Enter. If you press Shift+Enter, it will execute the current cell and we'll jump to the next one.
[00:02:53]
So as you can see, we just imported TensorFlow to our environment and we running TensorFlow 2.0, which been released just well, September 2019, right? So right now [LAUGH] it's about a month ago. All right, so that's the environment which we'll be using. And if you want to use JavaScript, I'm not gonna be using JavaScript, but you can try to simply clon this repository from tfjs-examples and use the provided examples from that repository.
[00:03:30]
All right, so let's go back, you have. So I've explained that we're gonna be using Whiteboards, Notebooks for the dependencies. So, for instance, I just demonstrated that I can import TensorFlow. To install TensorFlow on your machine, there are several ways how you can do it. You can just use pip install in the terminal.
[00:03:57]
So, for instance, if I have terminal open, I can do something like pip install tensorflow. And right now, it will just say that I already have TensorFlow installed. Or maybe it will even update it. No, it shouldn't. It should not. Yeah, I just basically check all the dependencies because TensorFlow, for instance, have NumPy dependency.
[00:04:24]
NumPy, by the way, it's numerical Python package. We'll be talking a little bit about it in a few minutes and other dependencies. And it will simply download it and install it on your machine. If you're using Conda package management, you should do those with Conda. So Conda install and then the package.
[00:04:46]
You can also do through the terminal, sorry, through the Jupyter notebook itself. So, for instance, let's just create new cell, Insert > Insert Cell Above. You can run a terminal commands in the Jupyter environment itself if you just put exclamation mark in front of it. So, for instance, !pip install tensorflow.
[00:05:14]
We'll execute these commands in your Jupyter server. So, if I once again gonna press Ctrl+Enter, you'll see that it's actually doing exactly the same thing. It's just checking all the dependencies and if the package is not installed, it will be installed and you can use it in your code.
[00:05:32]
So if, for instance, during executing any of my Jupyter notebooks you will see that import fails because of missing library, just do pip install and down correspond the library.
Learn Straight from the Experts Who Shape the Modern Web
- In-depth Courses
- Industry Leading Experts
- Learning Paths
- Live Interactive Workshops