Combining Neural Networks & AI
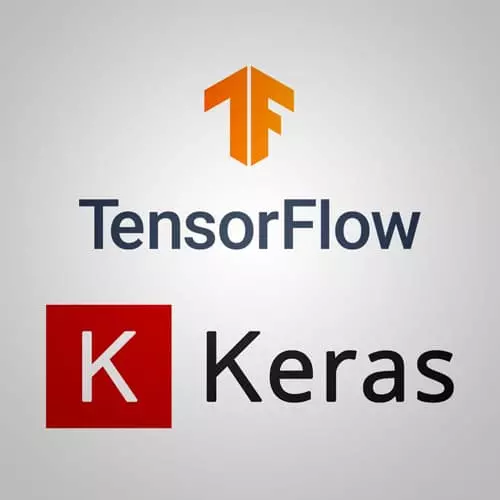
Check out a free preview of the full A Practical Guide to Machine Learning with TensorFlow 2.0 & Keras course
The "Combining Neural Networks & AI" Lesson is part of the full, A Practical Guide to Machine Learning with TensorFlow 2.0 & Keras course featured in this preview video. Here's what you'd learn in this lesson:
Vadim demonstrates how neural networks can be trained to work on different tasks, and have their work combined.
Transcript from the "Combining Neural Networks & AI" Lesson
[00:00:00]
>> Those topologies, those models they can learn a lot of things by just gonna doing that propagation, this training. And it's gonna now next level how you take those models and do something with them. For instance, another interesting example is combination of different neural networks. For instance, you can have convolutional neural network, which is used for processing images and some type of recurrent neural networks, which are used for data series, for instance, sound.
[00:00:34]
Waveform, right, is just the amplitudes or kinda change in pressure if we're talking physics in the air, but it can be just record it as your data series. You can do the prediction using convolutional neural network to basically see what is you're looking at on the image. And with select indications, you can even predict the location of those objects.
[00:01:03]
With RNN, so you can simply analyze text. And combining those together by mixing them together, what you can do, you can actually create the smart system which will analyze the question pretty used by user, right? So for instance just some question can be fit into RNN. And our model, so in this case, this model now is the combination of those simpler models, can try to give you an answer.
[00:01:33]
So for instance you can ask what color of the house I'm looking at. And CNN will analyze the image, will locate the house and will actually even find the color and the answer will be blue or brown, [LAUGH] or whatever. So you can kind of combine now those parts and pieces and the training will be similar.
[00:01:59]
You will need to provide a lot of questions, corresponding images and corresponding answer, what your model shoots give you as the results. So for instance, in the example I've used, it will just tell you blue, something like that. And with backpropagation, you will retrain convolutional neural networks and recurrent neural networks on the scan aggregator to figure out what should be the answers depending on the question.
[00:02:33]
So you can combine those parts and pieces. And for me, that is what I probably gonna call AI. So, yes.
>> So are these two different models and are they specializing in different-
>> Types of data?
>> Types of data?
>> Exactly.
>> So then could you have a model that let's say visualizes or can see, take camera and then a model that processes sound?
[00:03:00]
>> Yes, absolutely.
>> Like your eyes and your ears and another model that processes temperature.
>> Yes, so I put question here as the text but it can be sounds. Sound will be processed by recurrent neural networks You just need to have tons of training data. So it means that, for instance for this system, but then what should be our output?
[00:03:25]
What do we want to predict?
>> So let's say we're out in the world and we have hardware that can pull in that data and feed it in real time to the models, could predict whether that thing there's a duck based on the sounds and the-
>> Just the label but then you don't need the sound.
[00:03:47]
Although, yes if you wants to have almost like a system which will use additional source of information and in this case, sound as well, yes, absolutely. But I'm just saying that your system at the end will just produce the label, right, if we're looking at the dark or not dark.
[00:04:12]
But you can do so much more. So as I said, [LAUGH] no, I'm not talking about shooting the duck, we're not talking about automated robots killing stuff. It is possible but I'm against it, completely. Yeah, it's also deregulated, okay? But the thing is, yes, with all of those building blocks, you can almost mimic or replicate intelligence.
[00:04:44]
So answering questions, it's somehow in my brain, at least, associated with the intelligence. If you can answer my question, it means that he knows something, right? And this knowledge, understanding what first of all I'm asking you, and providing the answer yet behind the scenes it's just weights and biases.
[00:05:08]
But at the same time, those models starts, for instance, with text that I will show you. Somehow by still modifying with just those weights and biases, figuring out the relationship between objects in our world. So in word embedding, for instance, that's the trick used. If you kinda visualize this multi dimensional space, the distance and kinda the direction between two words, let's say, man and boy, will be the same as the direction between, for instance, woman and girl.
[00:05:53]
And just because the alignment, so somehow and once again, there are gonna be pretty cool demo there. Our system figuring out their relationship between different objects and it's learning by itself just by looking at the text. So yes, for me that's AI, the combination of multiple kind of building blocks originally more or less kinda simple ideas.
[00:06:20]
But now you can create something really incredible out of it.
Learn Straight from the Experts Who Shape the Modern Web
- In-depth Courses
- Industry Leading Experts
- Learning Paths
- Live Interactive Workshops