Bias in Training Data Sets
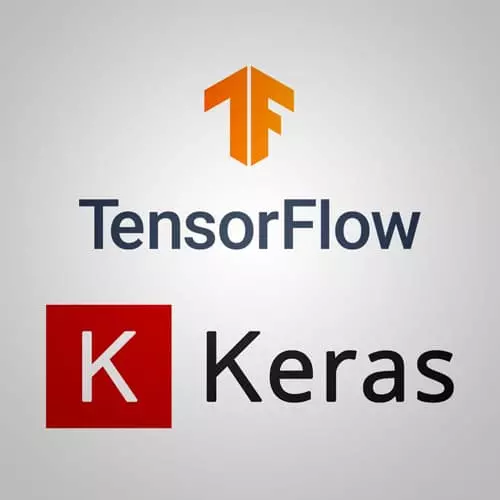
Check out a free preview of the full A Practical Guide to Machine Learning with TensorFlow 2.0 & Keras course
The "Bias in Training Data Sets" Lesson is part of the full, A Practical Guide to Machine Learning with TensorFlow 2.0 & Keras course featured in this preview video. Here's what you'd learn in this lesson:
Vadim explores training my the model into recognizing a new image, and focuses on where the attention of the neural network is.
Transcript from the "Bias in Training Data Sets" Lesson
[00:00:00]
>> So do we want to try anything else? Let's see creative common images. Let's grab the image address. So, JPG file, perfect. Okay, let's feed it into our neural networks. So, What we need to do, is simply download this image. So what I will do here is write the second command, ir maybe just create the new cell.
[00:00:40]
The location of the image and the image name will be the following. So all right, image downloaded, so now I can just simply put it as the name of our file. Seriously, I am doing for the first time and I have no idea what prediction we're gonna get.
[00:00:59]
So it should be pretty fun. Okay, so with the 36% accuracy, we are looking at the notebook, which is cracked, 29% laptop, and that was a mouse, really? [LAUGH] There's no mouse on the image, and that's actually really good example of biases in the data sets. So you see that our model actually did a prediction that it's looking at the mouse with yes, 8% not super accurate but still, it did this prediction.
[00:01:36]
And the reason why it did this because most probably a lot of images from the training data set had laptop and mouse on it. And that's kind of biases you probably want to avoid in your training data sets, right? When you have the almost like correlations between the particular classes.
[00:01:57]
And ideally what you want to have is equal distribution of laptops with mouses, laptops without mouses. Mouses without laptops, and of course, nothing, no mouse, no laptop. In this case, that's the balanced data set and what we want to have in your training data sets. But yes, it is working and we can even see okay, we need to change the index.
[00:02:24]
So the notebook, that was our neuron number 681. So everything is good, I just need to change this number. And now we can visualize the intensity, interesting, interesting, okay? Now, I'm kinda curious, if we overlaid those where the, [LAUGH] So when this prediction was made that we're looking at the laptop wasn't actually, well, it was partially looking the laptop, but it paid a lot of intention to the hands on the laptop, interesting.
[00:03:12]
So it's just one of the way to kinda play around with the potential where the neural network is. Where was the location of the neurons, which activated the prediction, which the neural network done. And it might help to avoid, as I said, biases or missed trained neural networks.
[00:03:36]
One interesting example was when there was a task to recognize dogs versus wolves. And well, they kinda look alike, right? And neural network was actually doing really good job predicting when it's looking at the wolves and when it's looking at the dogs. And when this method was applied we're gonna pay attention and figuring out where the neural network is looking at.
[00:04:01]
They figured that's when the image of the wolf was provided it was all the snow on the background. So neural network is just simply, it wasn't dog versus wolf predictor, it was simply telling you if it's recognized snow on the image or not. And if snow was present it was simply labeling all of that as wolf, is that it?
[00:04:20]
Well, you got the idea. But yeah, it's interesting and that's one of the way how you can see where the neural network is paying attention.
Learn Straight from the Experts Who Shape the Modern Web
- In-depth Courses
- Industry Leading Experts
- Learning Paths
- Live Interactive Workshops