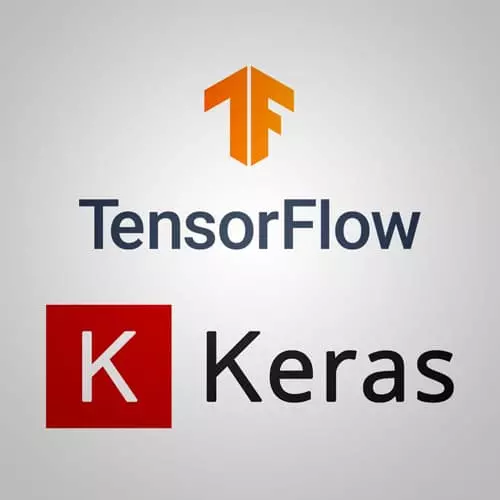
Check out a free preview of the full A Practical Guide to Machine Learning with TensorFlow 2.0 & Keras course
The "AI Modeling Demo" Lesson is part of the full, A Practical Guide to Machine Learning with TensorFlow 2.0 & Keras course featured in this preview video. Here's what you'd learn in this lesson:
Vadim highlights other examples where AI modeling changes the style of an image after being given data processed through deep learning.
Transcript from the "AI Modeling Demo" Lesson
[00:00:00]
>> That was the original picture. That's our style image and stylized image. Now you can see that you can kinda recognize the bridge right here. There's downtown right but all of those details are actually grabbed from the style image. So now we kinda can create something, right? And for me that is the representation of artificial intelligence.
[00:00:27]
And with AI now we can even synthesize ways in a video, video can be now created worth deep fakes, for instance, [LAUGH] A pretty well known example. So, AI is, for me at least all those kind of neat tricks which can help you to get closer to kinda creativity but still, it's somewhat scripted, right?
[00:00:54]
AI cannot completely create something from scratch, you still can have to use those building blocks to degrade something. Yes Micheal the next question.
>> Yes. So I get how you get the original image from the first layer because it's just the data that came in.
>> Yeah.
>> But then we're in that [COUGH] Process of recreating the image do you get the style applied?
[00:01:22]
I mean the style came from training the model.
>> All right let me switch to our diagrams and probably even start from scratch. So process, let's just draw it like this, right? Our sunglasses. So originally, let's take, let's say we took the style picture, right? And if you just take style picture so all right, now I think I know where the question is coming from.
[00:01:58]
The original model was trained on a lot of different pictures. So we used image net with those 1.4 million pictures and simply trained to recognize so we have nought zero Neuron but actually thousands of them, neurons. But it's still on the input we have pretty large pictures for instance, I know 256 by 256 pixels, right?
[00:02:25]
And it is reducing to thousands Pixels here and then deconvoluted back to the original size. But when we train our model to do that, it will recognize all those different objects right, so particularly neurons will be activated. But it will also learn this part right here with all the neurons, all the weight, how to recreate their original image, right?
[00:02:52]
So you can think about this model that it's not only learning how to based on the image, assign a particular label to it, but it also trying to figure out how to given a particular label recreate this object, redraw it. So that's what, for instance, that topology called, U-nex that's what it can do.
[00:03:22]
And what we doing next with this stylizing, right, so when we kinda have our model trained, we're doing forward propagation on the strange model. So it will just somehow analyze the image, right and kinda some of the neurons will be activated. And maybe we will even got some prediction here and it might gonna recreate this image back again, but we don't care about this part of the model at all.
[00:03:56]
We only care about the first few layers which grabbed the style of drawing. And why that exactly happening I will explain when we will talk about convolution narrow networks. But trust me on the first layers we are looking at kind of tiny details of particular colors, right? Have we diagonal lines there are vertical lines or something like that.
[00:04:23]
So we only care about this information, but when we do the photo, we actually do the full convolution deconvolution. So if we provided the image of the downtown at the ance, without any modification, we will reproduce the downtown image back again. But we can grab this layer, actually this layer, they are connected to each other.
[00:04:56]
So when I'm saying this layer was important because it layers it build a fact the weights in this layer. So we can just grab this part and substitute it into our photo. And that what will help our model instead of recreating the original image recreates the stylized image of the downtown, it's kinda conceptually what the neural network is doing.
[00:05:27]
But it's pretty cool you can actually play with it. I've included the source codes and all that is executable there, a couple of available other original images and stylized images. So, different monologue and Picasso paintings. So, you can play around with those, for instance. The only recommendation is trying to avoid original photos with a lot of tiny details.
[00:05:58]
Because this kind of information, the more details you have, those details will be ignored. So the most important parts, right, so kinda the big objects, what we see on the image, that will be propagated to the final result. But I think it's pretty interesting, right? And it's all about the information if you think about it like we started with the multi dimensional photo.
[00:06:22]
What is photo? It's like a huge amount of information. But we kinda reducing it to something and yeah that's what machine learning is doing, is basically doing something within the transformation, within the data, all right? And it's only doing is by figuring out what should be the weights and biases and connections between the neurons.
[00:06:42]
Yeah, that's another thing. Right now I mostly talking about it's really simple. The original kind of topology, fully connected neural networks when all the neurons connected from the previous layer to the next layer. But there are multiple modifications. For instance, Google come up with their architecture where they don't even have the symmetry.
[00:07:05]
They have kinda ad hoc branches which can do predictions by themselves, right? And that was actually the winner of the latest, not the latest but a couple years ago ImageNet competition.
Learn Straight from the Experts Who Shape the Modern Web
- In-depth Courses
- Industry Leading Experts
- Learning Paths
- Live Interactive Workshops