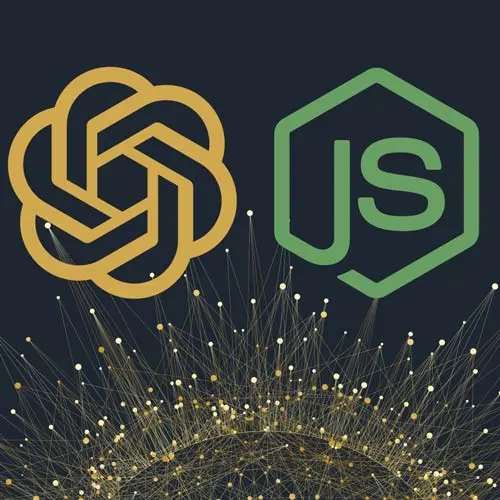
Check out a free preview of the full Build AI-Powered Apps with OpenAI and Node.js course
The "Performing a Similarity Search" Lesson is part of the full, Build AI-Powered Apps with OpenAI and Node.js course featured in this preview video. Here's what you'd learn in this lesson:
Scott codes the search function, which converts a query into an embedding and uses cosine similarity to compare it with other embeddings. The score of each search result is displayed demonstrating the proximity of each result.
Transcript from the "Performing a Similarity Search" Lesson
[00:00:00]
>> Okay, and then let's create the search function. So search, it's gonna be async. It's gonna take in a query, like that. And then we can take an account. This is like, how many results at most did you return? Let's default to 1. I don't know why I have two equal signs here.
[00:00:23]
That was weird. You have the count there. And then basically all we need to do is create a store, and we're gonna run this function here called the similaritySearch. So let's go back a second to, let's go back to this right here. Okay, so a similarity search, again that's like, okay, let's highlight this.
[00:00:47]
That's a different language. All these are different languages? No, that's okay. Okay, I'm like what is this? Okay, so let's say the query was, let me make that bigger. Let's say the query was, I don't know, trains, like, show me trains or something like that. Or, okay, this result might come back because it mentioned something about a railway, right?
[00:01:17]
That's the semantics behind that. And, yeah, so in order to do that, I need to take the query that you are sending and I need to throw it in here. I have to throw it in here, otherwise, I can't do math on how close something is to it.
[00:01:35]
So just like we are taking all these documents and using this embeddings function to convert them to embeddings, which are a list of vectors which are just an array of numbers, we have to do the same thing with the query. The query also needs to be converted to a list of numbers so we can throw it in the same plot as these numbers, and compare them to see how close they are to each other, right?
[00:02:01]
So like, let's say that train lands here. Okay, if I had a train right here on this mouse, this is really close to it. So let's return that, right? So that's how that works. Okay, so let's do that. So const store = await createStore() This is gonna create our store which indexes our documents, which creates embeddings for them.
[00:02:30]
So now we have those. We have embeddings here, that's done. The next thing we need to do, like I said, is this similaritySearch. There's many different algorithms that calculates simulators very differently. I don't think I can even talk about them all there, just beyond me. But one of the most popular ones is the cosine similarity search.
[00:02:56]
If you wanna read about that, go read about it, but that's basically what this simulator search is going to be doing. It's just doing math on these different vectors and how close they are. So that's the search that we're gonna do. There's so many different types of them, but I think that's what we get for free when we call a similaritySearch.
[00:03:13]
So what we're gonna do is return store.similaritySearch. We can actually even do a similarity search with a score, which will return them ranked by how relative they were to the query. So that's useful, so you can sort them on your UI, like this is the one that's most likely versus not, so you can do whatever you want there.
[00:03:37]
We're just gonna do a similaritySearch. We're gonna pass in the query, And then, how many at most we want back? So what the similaritySearch is gonna do, it's gonna convert this query into an embedding, just like this is an embedding. It's gonna perform that cosine similarity search to see which one of these embeddings are closest to this embedding.
[00:04:05]
And it's going to return all the matching documents that are closest to that. Does that make sense? Okay. Cool and then the last thing we need to do is just search anything here, right? So let's try that. So I'll go on the next line, and I'll say console.log.
[00:04:29]
I'm going to await my search. And then let's search for something, so we got some movies here. I want to, A movie that will make me feel like, I am crazy. [LAUGH] A movie that will make me feel like I'm crazy, right? That's the type of movie I wanna find, I'm in for that.
[00:04:55]
Because actually I do movies that make me feel like, okay, I doesn't like some of the best movies. So if this works, let's try to run it. So we'll say search, and you see, I get back Shutter Island. Yeah, that movie is definitely gonna make you feel absolutely insane, right?
[00:05:13]
And you can see here's the metadata associated with it, and here's the title, right? How did it know that? Well, I mean, it says it's a mind bending plot. So yeah, that's probably gonna make you feel crazy, right? So, let's try another one. I have Paw Patrol here, right?
[00:05:28]
So if I wanna say something cute and fluffy, right? Something cute and fluffy, I don't think I mentioned that anywhere in here. There's nothing cute and fluffy. I mean, there's no word of cute-
>> Little puppies.
>> Anywhere, right? Just puppies, right? So let's see. I would imagine Paw Patrol is gonna come out because all the other movies are like pretty intense in that list.
[00:05:54]
Yeah, Paw Patrol does come back, all right? That's pretty cool. You could not make something like this. I don't know, just two years ago, this simple, it's just very simple to be able to do that. And again, remember at its core, we're just comparing numbers together. That's it.
[00:06:14]
These things get turned into numbers. This query gets turned into numbers, and we're seeing which group of numbers are closest to this group of numbers when we plot them out. That's how that works, yes.
>> And how does the count factor into that result?
>> Yeah, count just, yeah, it's just like how many you want returned.
[00:06:35]
So if I put three, I guess it's gonna go try to find, I don't know, two more movies. I mean, it'll return all the movies, but it'll return them in order of which is the closest. So if I put like 4, it'll give me a list of the four closest movies, right?
[00:06:53]
So I said, cute and fluffy, so Paw Patrol was the strongest. Then stepbrothers, which is a funny movie, then interstellar. Why did it say that? I don't know. And then Shutter Island. So yeah, I have something in here for like, I'm like, how did it know? Because I guess this could be like cute and fluffy, cuz if you've seen this movie is like a brown family and stuff.
[00:07:17]
I'm like, I didn't put that in there. So I was like, I got scared for a little bit when they put that in there. But it doesn't mean that it's related, it's just like that's the third closest one, it could be unrelated. But because I said give me four it had to give me four.
[00:07:31]
So even though it might be all the way over there, that's the fourth closest one. So that's why I came back
>> Small dataset problems
>> Small data set problems. Exactly, yeah. Cool, which is something you got to like account for and like yeah, there's there's. So that' why I like doing the one with a score.
[00:07:49]
>> Yeah, the score would help that.
>> Exactly, you do like that swamp with a score here. You can filter out the ones that don't have high enough score. You'd be like, you know what? If you might do like a delta, okay, grab the score from the highest scoring one.
[00:08:06]
And then anyone that is of delta less than, some percentage or some value from the highest one, exclude them. They're just too far. They're just way too far. So what is the difference between this and the second one? Because you might go from here and then you go here, and it's just like, okay, that's a big drop.
[00:08:25]
Like, what is this? That's a whole point right there, right? This one from 77 to immediately 730. That tells me that like, this is nothing like this one is such a big job. So I will probably just exclude these, cuz the difference between these two is too high, right?
[00:08:44]
There's a gap there, so that's like one way. But it's like all these little things that you have to do to account for that? I mean we did this and 59 lines of code, if you just got rid of the movies, [LAUGH] it's just like two functions it's just this that's it.
[00:09:04]
That is insane to me that that's all it took for me to be able to do this. So, yeah, I can't wait to see what people build when everything just has all this stuff in it. So it's quite impressive.
Learn Straight from the Experts Who Shape the Modern Web
- In-depth Courses
- Industry Leading Experts
- Learning Paths
- Live Interactive Workshops