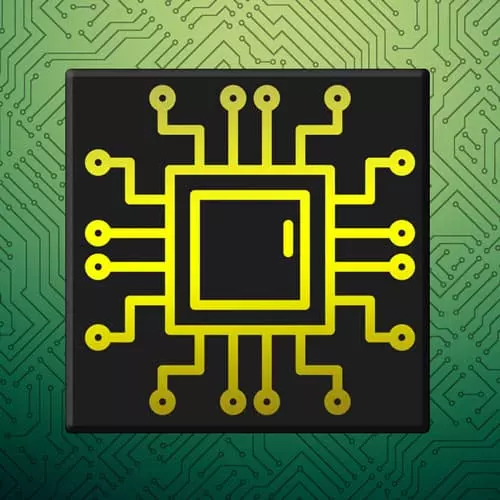
Check out a free preview of the full Complete Intro to Computer Science course
The "Quick Sort Q&A" Lesson is part of the full, Complete Intro to Computer Science course featured in this preview video. Here's what you'd learn in this lesson:
Brian answers students questions regarding an error involving missing the - 1 in nums.length -1, if overwriting the variables would improve the memory, how to use a for of loop to solve the example, and walks through of the time complexity of this code. Student questions regarding how to decide what the log(n) is, if it makes sense to choose pivots outside the data set, and if choosing the perfect pivot is worth it are also covered in this segment.
Transcript from the "Quick Sort Q&A" Lesson
[00:00:00]
>> I have a quick question about an error that I cause
>> Sure.
>> When I first made it, I forgot to put the in for loop, the number dot length minus 1. And basically it seemed to cause a stack overflow and I was wondering I was trying to figure out why that would cause that.
[00:00:18]
And I was wondering if you could give me some idea.
>> Sure, so he's saying he forgot to put the minus 1 here and that was causing a stack overflow.
>> That's it looks like, yeah.
>> Because so it means you're inserting your pivot into the left and right.
[00:00:35]
It probably means that eventually, you're gonna run into an array that's always of link 2, right? So it's going to keep calling that. Right array or left array, which is never gonna hit that base case, right? Because it's never gonna hit link one, because those arrays have to get progressively smaller and smaller.
[00:00:55]
>> Okay, and why would it be stuck at 2?
>> So let me see if I can invent an example. I think if you did this where your number is equal to 1, let's do 9, 8, 7, so 7 would be the pivot And then it would try and break this down and 7 would always get.
[00:01:27]
So basically, this would just keep calling recursively endlessly on 9 and 8, 9, 8, 7, assuming everything went into the right array. Or 9 or so maybe this is better, Because everything would always go into the left array. No, I had a right before okay. So seven Because this is not equal to this or this is equal to this rather everything would always get put into the right array.
[00:01:59]
So then it would call quicksort on right which would always endlessly be 9,8,7.
>> Yeah I have chosen the question from movement of especially convexity, and when we call quicksort, left and quicksort, right, if we just our wide left and right array, so we don't create a new one every time.
[00:02:23]
So maybe it's whatever improve the spatial complexity.
>> Specifically here, he's asked, I think you're asking me online set 37, 38 instead of storing these variables, what if we just overrode them? The amount that you would affects the memory would be trivial to the point that I say it wouldn't matter.
[00:02:43]
Because sort of left and sorted, these are actually just gonna be pointers. They're actually not gonna be full copies and pointers are the smallest thing you can possibly store in memory. So that would be a optimization that I don't think would really help you very much. Might help a little bit.
[00:03:01]
But it's like grabbing a bucket out of the ocean, right? It's gonna be such a small amount versus the much bigger things that are going on.
>> I tried to be smart and use for off instead of for loop. And I had the same problem essentially right? I wasn't removing pivot and I were just stuck.
[00:03:23]
>> Yep, so he's saying instead of using a for loop he used like the functional like for each or something like that.
>> For off.
>> For off loop yeah, so a for off loop here which looks like for lead item of numbers like this right? The unfortunate thing about this is, it's always going to capture the last item in the array, which means your pivot is gonna be put into your left or right, which means that you're gonna probably end up in a stack overflow situation.
[00:04:00]
So a lot of just normal for loops. I know they're not the most appealing looking piece of code, but they are extremely useful.
>> Poke the pivots and use the for off without problem.
>> Yep, so you could absolutely just say, pivot equals instead of doing this, he would say const pivot equals nums dot pop right and this would actually remove the pivot from the from the array and then you could absolutely do a for of loop here.
[00:04:39]
That would work great. Many ways to skin this cat.
>> Can you please walk us through the time complexity of this code?
>> Yeah, so the time complexity of this is average case scenario and login. So let's look down here a unit test case. So here, we're gonna put 5 as the pivot and then, 10,8,6,9,7 are gonna go on the right array and then everything else is gonna go on the left array.
[00:05:22]
So this is to say every item is going to be looked at the at least once, right because we're splitting it into different pieces. So let's just say that there's at least n. So that's where the n part of this is because we're gonna look at everything at least once and make comparisons at least once for everything.
[00:05:38]
However, when we're doing the yeah, so when we're putting 7 into the right array and we're putting 4 into the left array, 4 and 7 are actually never going to be compared to each other ever. We're doing this by virtue of the fact that if we know something is smaller than 4 and we know something is larger than 5, right?
[00:06:03]
That means that we can make the fundamental assumption using the transitive property. And I don't know I did air quotes there. It's literally called the transitive property in mathematics. 4 must be smaller than 7, right? So we can use that property of mathematics to say like cool. 4 and 7 never have to be compared to each other because I already compare them both to 5, right?
[00:06:24]
So that means that we're going to get these like economies of scale. So if we do a huge quicksort on a huge array, that means that there's a bunch of comparisons that we never have to do, as opposed to something like insertion sort, which where everything has to be compared against everything else every single time an average case scenario.
[00:06:45]
That's why that one ends up being n squared and this one, it's average case scenario ends up being n log n because normally they don't have to be compared to each other because we can look at those pivots. So that's why it ends up as n log n.
[00:07:00]
>> Yeah, but there's something I'm struggling with. How could I get to the login part from the code? So I guess maybe mathematically, help me understand it better. But yeah, I understand that it's because it's a divide and conquer algorithm is getting smaller and smaller and we're working with the smaller lists but getting to that login just by looking at the code i.e, I'm struggling with that.
[00:07:35]
>> Unfortunately there's no and hopefully answering your question that you have. Like you there's no hack here for us to look at and say, we have nested for loops, therefore we have n squared, right, unfortunately, there's not really a good way of doing that here. The best indication that you have this is gonna be log in is that it's recursive.
[00:08:00]
But still that's that's an indication it's indicates to you that it might be login. But it's not necessarily like we saw, for example that count two or factorial for example, those are not, those are just n, right? They're not login. So the way that you have to conceptualize in your head to figure out what the login is, is that just what I was telling you before those diminishing the more items I throw out this, the less comparisons that it adds.
[00:08:32]
As we add more we get those kind of economies of scale. Once you kind of realize that like you're using these kind of transitive properties to avoid making some of the comparisons, that's where it's gonna say like okay, this is definitely login right that's the indication that's the proof that you need in your head for you to say this is login because I don't have to compare everything to everything else.
[00:08:54]
I don't really know if I have a more succinct answer than that. Yeah, it, it's hard [LAUGH] And like even when I'm sitting there, like I've messed this up in interviews as well, I remember specifically that I messed this up in a Facebook interview. So it's not apparent and it's pretty difficult, right?
[00:09:15]
Which is probably why they choose to ask you these questions.
>> Assuming we have some data set that we know, a statistical distribution about, does it make sense to choose a pivot with outside the data sets? Like okay, I these are student grades and I know the they are in the range of 4200 usually and the mean value is about 70, so I can start with 70 without actually including it.
[00:09:53]
Just for the first iteration, does it make sense?
>> So the question is if you have some knowledge of the distribution and the average and the median, perhaps, maybe the medians rather than most, actually, it's definitely the most useful thing there. Would you be better off selecting something that you know to be a good pivot that might be outside of the normal data set.
[00:10:25]
I guess, yeah, I can see that definitely being helpful. And just not including the pivot, right, cuz the pivot wouldn't be actually in the data set. That's only gonna help you for the first pivot though, right? And the disadvantage that we're gonna have here is, now we have to have a specialized case that the first level of recursion is gonna be different than every other level of recursion, right?
[00:10:48]
Because at that point, once we go down into the lower level of recursion, all bets are off, we can't use the same pivot again.
>> I was thinking like since we are already iterating over everything in the first pass, maybe while we're sorting it into left and right parts we could be Just taking a running average or something like that?
[00:11:16]
>> So,
>> For the next pivot, I mean
>> The issue with like, you could always like, you could write a piece of code to always select the perfect pivot, right? But then when you're looking at every item in the array every single time, which greatly adds to the complexity.
[00:11:34]
[LAUGH] So that that in and of itself, always looking for the perfect pivot, you're actually ruining what makes this algorithm efficient at all right? Which is the fact that you don't have to look at every item every single time. In the sense of, yeah, that you would have to first find the perfect pivot.
[00:12:01]
Yeah, and then that would kind of ruin the fact that you, you had to search through at once to find the median, pivot and then split it. I think the thing that points to after that is like there are more efficient ways of selecting that pivot. The one that I'm familiar again is Quicksort.
[00:12:19]
Three, we look at the first item, the middle item and the last item. And that that mostly solves almost all of the distribution problems that you'd run into And that's kind of the one that people tend to favorite because one, it's fairly simple and easy to understand and to it it like, prevents you from having worst case scenarios.
[00:12:40]
But there are some other ways of selecting that pivot that I'm actually not familiar with submit one of them might do that. I just can't conceptualize a pyramid off the top, my head.
>> So very simple sample just source 80% of the problem maybe.
>> Yeah, you could still have the problem where you were always selecting the top three items in the array But the statistical probability of that is not very large, right?
[00:13:11]
Cuz if you're always selecting the bottom three or the top three items in the array you still run into the worst case scenario. Which is not great, but the fact that you go from doing that frequently with just one pivot to almost never if you select three pivots and then choose the median one.
[00:13:31]
>> It's kind of complicated depending on data set. Maybe there are some specific cases where just the guessing a good pivot would make sense. But I think what you say covers so much ground just by itself. That's what I said, it probably becomes unnecessary in most cases?
>> Well, and I think another thing that you might be thinking, you should think about is if you have that much knowledge about what your dataset is gonna look for, or is what it's gonna look like, quicksorts' probably not the best choice at that point, right?
[00:14:06]
It might be like something insertion sort, cuz if it's already nearly sorted, then insertion sort is gonna be better. And if you already know the median, I guess if you know the median and you know it's totally shuffled, maybe. [LAUGH]
>> I mean, student grades are a good example.
[00:14:21]
You know the distribution, but they may come in any order.
>> But at that point, if it's gonna come in any order quicksort is already very good at that. And having the perfect pivot doesn't make it a whole lot more effective, right? As long as it's an okay pivot.
[00:14:39]
It usually works pretty well.
Learn Straight from the Experts Who Shape the Modern Web
- In-depth Courses
- Industry Leading Experts
- Learning Paths
- Live Interactive Workshops